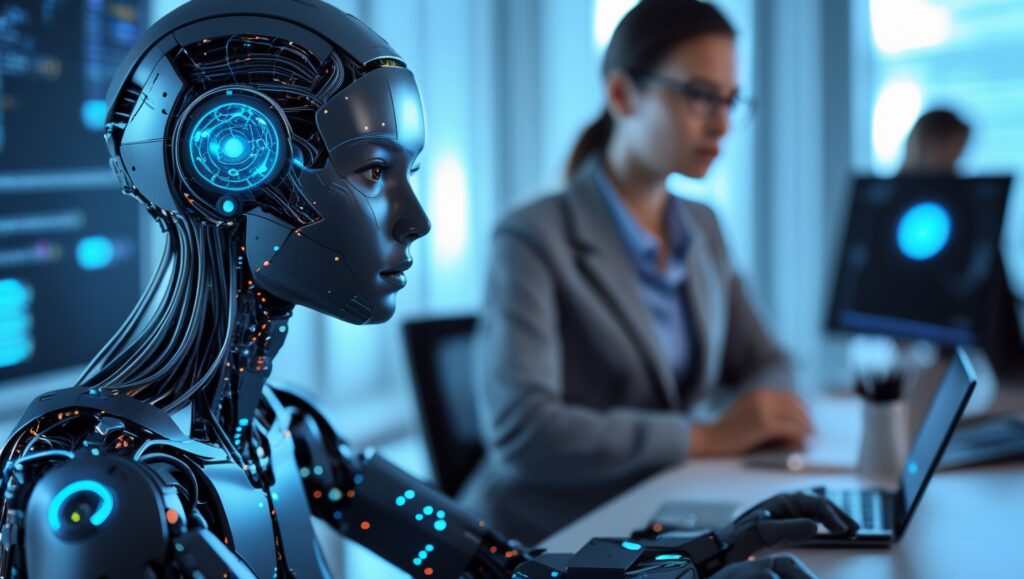
The Stakes of AI Ethics
Artificial Intelligence (AI) is no longer a futuristic concept; it is deeply embedded in our daily lives. From healthcare diagnostics to criminal justice, AI systems are making decisions that have profound real-world consequences. However, the rapid advancement of AI technologies has outpaced the development of ethical guidelines, leading to significant societal challenges. The stakes of AI ethics are high, as algorithmic decisions can perpetuate bias, invade privacy, and even endanger lives. Ethical considerations cannot be an afterthought; they must be integral to the development and deployment of AI systems. This tension between innovation and responsibility is at the heart of the AI ethics debate.
Core Ethical Principles in AI
Core Principles of AI Ethics
Transparency and Explainability
AI systems should be transparent, and their decisions should be explainable to users and stakeholders.
Fairness and Non-Discrimination
AI systems must be designed to avoid bias and ensure fairness, preventing discriminatory outcomes.
Privacy and Data Protection
Protecting user data is paramount. AI systems should comply with data protection regulations.
Safety and Security
AI systems must be safe and secure, minimizing risks of harm to users and society.
Human Oversight and Governance
Human oversight is essential to ensure AI systems operate within ethical boundaries.
Case Studies in AI Ethics
Healthcare Algorithm Bias
A notable example of bias in AI is the case of a healthcare algorithm used in the United States to allocate care to patients. The algorithm was found to favor white patients over black patients, leading to significant disparities in healthcare access. This bias stemmed from the use of historical healthcare spending data, which reflected existing inequalities.
Facial Recognition Controversies
Facial recognition technology has been criticized for its inaccuracies and biases, particularly in identifying individuals from minority groups. These inaccuracies have led to wrongful arrests and heightened surveillance concerns, raising questions about the ethical use of such technologies.
Language Model Biases and Misinformation
Large language models, like GPT-3, have been found to generate biased and harmful content. These models can perpetuate stereotypes and spread misinformation, highlighting the need for ethical guidelines in their development and deployment.
Autonomous Systems Decision-Making
Autonomous vehicles and drones face ethical dilemmas in decision-making, such as the “trolley problem.” These scenarios require careful consideration of moral principles and the potential consequences of AI-driven decisions.
Stakeholder Perspectives
AI Ethics Case Studies
Healthcare Algorithm Bias
A notable example of bias in AI is the case of a healthcare algorithm used in the United States to allocate care to patients. The algorithm was found to favor white patients over black patients, leading to significant disparities in healthcare access. This bias stemmed from the use of historical healthcare spending data, which reflected existing inequalities.
Facial Recognition Controversies
Facial recognition technology has been criticized for its inaccuracies and biases, particularly in identifying individuals from minority groups. These inaccuracies have led to wrongful arrests and heightened surveillance concerns, raising questions about the ethical use of such technologies.
Language Model Biases and Misinformation
Large language models, like GPT-3, have been found to generate biased and harmful content. These models can perpetuate stereotypes and spread misinformation, highlighting the need for ethical guidelines in their development and deployment.
Autonomous Systems Decision-Making
Autonomous vehicles and drones face ethical dilemmas in decision-making, such as the “trolley problem.” These scenarios require careful consideration of moral principles and the potential consequences of AI-driven decisions.
Practical Approaches to Responsible AI
Ethics by Design Methodologies
Incorporating ethical considerations into the design phase of AI systems can help prevent issues before they arise. This involves interdisciplinary collaboration and continuous ethical assessments.
Testing and Evaluation Frameworks
Rigorous testing and evaluation are necessary to identify and mitigate biases in AI systems. Frameworks like the AI Fairness 360 toolkit provide tools for assessing fairness in AI models.
Diverse Development Teams
Diverse teams bring varied perspectives, which can help identify and address potential biases in AI systems. Inclusivity in AI development is crucial for creating fair and equitable technologies.
Feedback Mechanisms and Redress
Establishing feedback mechanisms and redress processes allows users to report issues and seek remedies. This promotes accountability and continuous improvement in AI systems.
The Future of AI Governance
Emerging Standards and Frameworks
Emerging standards, such as the IEEE’s Ethically Aligned Design, provide guidelines for ethical AI development. These frameworks aim to harmonize global efforts in AI governance.
Self-Regulation vs. Government Oversight
The debate between self-regulation and government oversight continues. While self-regulation allows for flexibility, government oversight ensures accountability and enforcement of ethical standards.
Global Coordination Challenges
AI governance requires global coordination to address cross-border issues and ensure consistency in ethical standards. International organizations like the United Nations are playing a role in facilitating this coordination.
Individual Agency in an Algorithmic World
Empowering individuals with knowledge and tools to understand and challenge AI decisions is crucial. This involves promoting digital literacy and ensuring transparency in AI systems.
Ethical AI Assessment Framework
To help readers evaluate AI systems, here is a simplified “Ethical AI Assessment” framework:
- Transparency: Is the AI system’s decision-making process transparent and explainable?
- Fairness: Has the system been tested for biases and discriminatory outcomes?
- Privacy: Does the system comply with data protection regulations and ensure user privacy?
- Safety: Is the system robust and secure against potential risks and failures?
- Human Oversight: Is there adequate human oversight and governance in place?
Who’s Responsible?
Different stakeholders have varying responsibilities in ensuring ethical AI:
- Developers: Must design and test AI systems with ethical considerations in mind.
- Companies: Should establish ethical guidelines and ensure compliance.
- Regulators: Need to create and enforce regulations that promote ethical AI.
- Civil Society: Must advocate for the protection of human rights and social justice.
- Users: Should be informed and vigilant about the AI systems they interact with.
External Resource Recommendations
- Academic Ethics Frameworks: IEEE Ethically Aligned Design
- Industry Ethical Guidelines: Google AI Principles
- Civil Society Position Papers: AI Now Institute Reports
- Government/Regulatory Documents: EU AI Act
- Tools for Ethical AI Assessment: AI Fairness 360 Toolkit