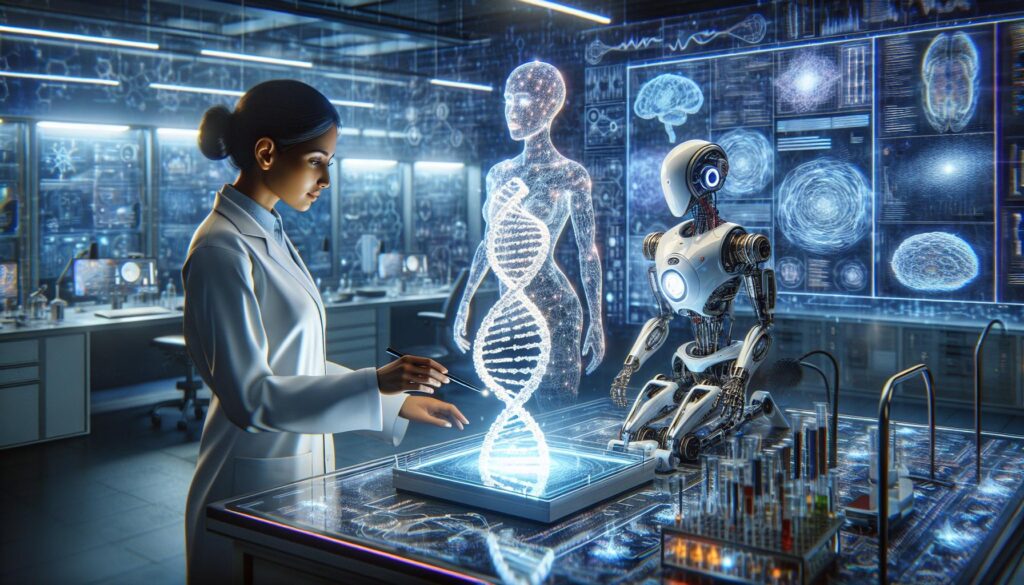
When Google unveiled its AI “co-scientist” in early 2025—a multi-agent system developed on its Gemini 2.0 platform—many in the tech world saw it as a bold leap toward automating discovery. But among leading researchers, the reaction was more measured.
“AI holds great promise but remains a tool—researchers’ insight, judgment, and creativity cannot be replaced,”
noted several experts interviewed by KUNC News.
Why? Because while AI can process more data than a human could in a lifetime, it still can’t grasp the intuitive leaps, ethical nuances, or experimental rigor required for real scientific progress.
AI as a Catalyst, Not a Creator
Artificial intelligence is making waves in labs across the globe—not by delivering Eurekas on its own, but by supercharging how humans explore complex problems. AI tools today are being used to scour decades’ worth of journal articles in seconds, formulate potential hypotheses, and even flag anomalies in experimental data. For example, Google’s AI co-scientist system is designed to “uncover new, original knowledge and to formulate novel research hypotheses,” explained Google Fellow Juraj Gottweis in a blog post on the project.
This isn’t science fiction—it’s already in action. According to the 2025 AI Index Report by Stanford HAI, machine learning models are now involved in over 70% of biomedical research pipelines, helping scientists identify viable drug targets and streamline clinical trials. But here’s the rub: these tools still depend heavily on human oversight. Without expert validation, such models can hallucinate plausible-sounding but false conclusions—a serious risk in something as sensitive as medicine.
Where AI Shines: Cross-Disciplinary Insights
One of AI’s most exciting strengths lies in its ability to connect dots that humans often overlook—especially across traditionally siloed disciplines. Think about it: what happens when data from climatology, genomics, and materials science are analyzed simultaneously? AI can help detect patterns or correlations ripe for further investigation, potentially leading to hybrid fields or unforeseen breakthroughs.
Take, for instance, an emerging collaboration between AI systems and climate scientists at the Lawrence Berkeley National Laboratory. By integrating atmospheric chemistry with machine learning tools, researchers have identified previously unknown pollutants interacting with greenhouse gases—an insight that would have taken years without AI support. These interdisciplinary leaps are redefining the nature of discovery, but they still require humans to formulate the right questions.
Limits of the Algorithm
Despite all the excitement, AI’s track record in research isn’t flawless. A peer-reviewed NIH article from 2024 outlines a few key concerns: bias amplification, questionable authorship ethics, and data inaccuracies.
“The integration of AI into research raises questions of data integrity and the potential propagation of biases.”
Plus, there’s the issue of what some call “experimental hygiene”—a term describing the rigorous standards scientists use to verify results. AI can suggest hypotheses, but it can’t run lab experiments or ensure that the data isn’t skewed by poor methodology. Hypotheses without reproducible proof are just guesses, no matter how sophisticated the algorithm.
Opening New Scientific Doors for All
There’s also a compelling upside to AI in science that doesn’t get enough airplay: democratization. AI-powered research tools could empower underfunded institutions or researchers in developing countries by providing access to computational muscle that would otherwise be out of reach. This trend is creating new possibilities for scientific inclusion, as noted in the MIT Sloan article and echoed throughout the tech industry.
Platforms trained on open-access data can now assist researchers in places with limited lab infrastructure, leveling the playing field in ways traditional grant systems never could. Still, as highlighted in the AAAI Presidential Panel on the Future of AI Research, responsible development and accessibility must go hand-in-hand to avoid deepening existing inequalities.
The Tech Under the Hood: Future-Ready Science
With AI’s appetite for processing power growing exponentially, emerging computing technologies are racing to keep up. Tools like quantum and photonic computing are being tested as platforms better suited to handle the complexity of scientific AI tasks. According to Morgan Stanley’s 2025 Tech Trends report, these next-gen architectures could enable AI models to reason at levels currently beyond today’s silicon-based systems.
That means we aren’t just advancing scientific questions—we’re transforming the very tools used to answer them. The partnership between humans and AI is becoming more like an ecosystem than a workflow. And that shift could reshape what, how, and who discovers the next big thing.
The Bottom Line: Augment, Don’t Automate
AI isn’t your next Nobel Prize winner—but it might help you win one. While the headlines often pitch a future where AI “does the science,” top researchers continue to emphasize that creativity, ethical discernment, and scientific intuition remain fundamentally human traits. AI is just another instrument—albeit an extraordinary one—in the scientist’s toolkit.
So as you read about AI’s growing capabilities in science, remember: the real breakthrough isn’t that a machine can think like a scientist. It’s that it can empower more humans to do science better, faster, and—perhaps most importantly—together.
Conclusion
If the future of science is a shared endeavor between human minds and machine intelligence, then the real question isn’t whether AI can replace scientists—but whether we’re prepared to redefine what it means to be one.
Can we imagine a research landscape where insight comes not just from solitary genius in white lab coats, but from collective symphonies of algorithms, data, and human curiosity woven together across continents and disciplines?
This vision invites a rethink of more than just scientific tools—it challenges the traditional gatekeepers of knowledge, the meaning of authorship, and even the pace at which discovery unfolds. As AI reshapes not only the answers we get but the questions we dare to ask, the next big breakthrough may not come from a single lab or machine, but from a radical shift in how—and who—we trust to explore the unknown.