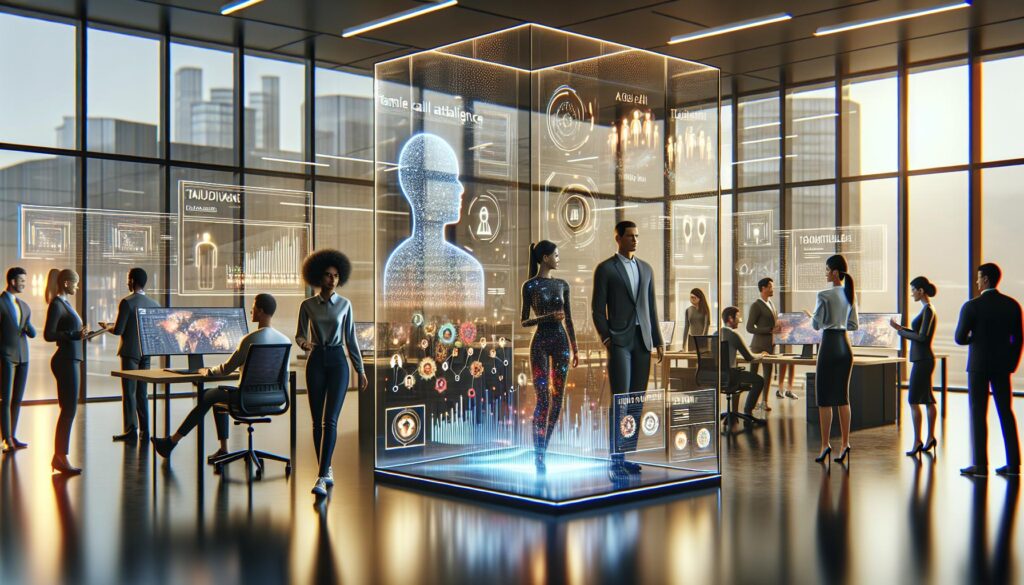
At UPS, an internal pilot program recently used AI to analyze how delivery routes, package weight, and weather disruptions impact team performance. The outcome? An 18% efficiency increase—not by replacing workers, but by better coordinating what’s already in place. This isn’t about outsmarting talent; it’s about outworking inefficiency. Welcome to the rise of work intelligence.
From Individuals to Ecosystems
Traditionally, managing talent has been about spotting top performers, nurturing their growth, and holding onto them as long as possible. This idea, known as talent intelligence, is rooted in data-driven approaches to hiring and development—what skills a person has, how they perform, and where they fit in the organizational puzzle. Platforms like Beamery and Magnit help companies use data to make smarter people decisions.
But here’s the twist: work intelligence flips that focus. Rather than spotlighting individuals, it looks at the performance of the entire system—teams, tools, processes, and yes, the interactions between them. Exec explains this as the shift from human capital to organizational capability: how well all the parts work together.
According to a CADS.ai report, the average Fortune 500 company now has over 250 different job roles with overlapping skill sets. Is it more useful to map the top developer—or to understand how developers, data analysts, and customer service teams work best as a unit?
AI Makes It All Possible
This shift couldn’t happen without a technological backbone. That’s where AI and data science come in. AI can not only analyze resumes or screen applicants—it can map workflows, pinpoint communication gaps, and recommend real-time adjustments.
Eightfold.ai gives a great example: HR teams using AI to evaluate not just who has the right title, but which employees have skill adjacencies—hidden capabilities that could be trained up within weeks, not years. That insight is gold when you’re hiring in a fast-moving market.
And here’s a surprising stat: According to Coursera, AI-enabled data science models can parse 500+ organizational data points per minute, compared to a human analyst’s 100 per hour. That’s not replacing intuition—it’s amplifying insight.
Real-World Applications
Here are a few places where work intelligence is already changing the game:
- Retail: A global apparel brand used AI to assess how store layouts impacted team dynamics and sales conversion. The result? A 12% boost in revenue per square foot, without adding staff.
- Tech: At a major software firm, AI matched cross-functional skills between engineering and marketing teams to create multi-skilled agile pods. The company cut product release cycles by 30%.
- Healthcare: Hospitals are leveraging work intelligence to optimize scheduling around staff fatigue and patient loads. Smart systems help avoid burnout while improving patient outcomes.
These examples show one key truth: Good talent matters—but how that talent works together matters more.
The Broader Tech Wave
This movement doesn’t exist in isolation. It rides the same wave as edge computing, GPT-based copilots, and cloud-native platforms. As Josh Bersin aptly notes:
“The future of work isn’t who works—it’s how work is done.”
As AI integrates with tools from Magnit and Coursera, organizations will stop asking, “Who can we hire?” and start asking, “How can we optimize the work itself?”
What Comes Next?
Don’t be surprised if your next performance review includes data points not just about what you accomplished—but how your work multiplied the productivity of your team. Not because someone’s watching you, but because smart systems are now built to understand those ripple effects.
As the lines between people, tasks, and tech continue to blur, AI won’t just help companies hire better—it will help them work smarter. Talent will always matter, but in the coming decade, those who understand work intelligence will have the real edge.
So the next time someone asks whether AI is replacing jobs, flip the script: It’s not about replacing jobs—it’s about replacing unchecked assumptions with intelligence that scales.
Conclusion
If AI can now see how work unfolds—not just who does it—are we looking at the workforce all wrong? Maybe it’s not about finding brighter stars, but about lighting up the whole constellation. As AI weaves deeper into the fabric of our processes, the big question isn’t whether machines will do our jobs—it’s whether we’ve truly understood what “doing the job” even means in a networked, intelligent system.
This shift reframes more than strategy; it reframes responsibility. What if future breakthroughs won’t hinge on lone genius or polished résumés, but on how well people, processes, and platforms align in real time? In this light, work intelligence isn’t just another trend—it’s a prompt to rethink the very architecture of how value is created and shared. The tools are here. The insight is growing. The only question now is: are we ready to work differently?