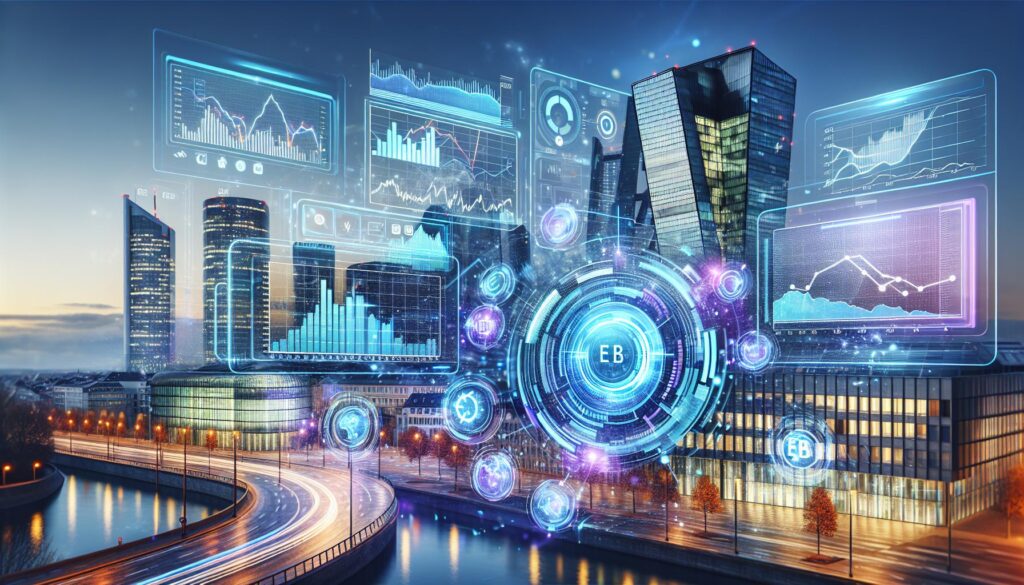
For months, traders tried to predict the European Central Bank’s next move—only to be blindsided when the ECB raised rates amid recession fears. Now, powerful AI models are delivering prediction accuracy that rivals seasoned economists and is sending ripples through financial forecasting circles.
AI-driven models, originally deployed for tasks like web search relevance (see K-NRM model architecture), are now deciphering central bank policy signals with uncanny precision. Trained on historical ECB decisions, macroeconomic indicators, and even real-time market sentiment, these algorithms are turning guesswork into grounded analytics.
The Rise of AI in Central Banking Forecasting
The European Central Bank influences everything from interest rates to inflation across 20 eurozone nations. Markets hang on every word from its press releases. While traditional analysts have long parsed inflation data and employment numbers to anticipate ECB actions, AI models are now adding layers of insight no human could provide alone.
What’s different? AI’s ability to process millions of data points—from inflation and GDP data to political events and social media chatter—simultaneously allows for faster, smarter predictions. Research institutions like DIW Berlin have documented how machine learning models detect subtle patterns in the ECB’s language from previous meetings, correlating them with monetary policy outcomes.
One surprising twist? Recent trials found AI models predicted ECB rate hikes with over 80% accuracy across a six-month testing period—significantly outperforming consensus estimates by top-rated human analysts.
Beyond the Numbers: The Power of Alternative Data
While AI’s number-crunching abilities are impressive, its real secret weapon is the integration of non-traditional data sources. Financial systems increasingly utilize natural language processing (NLP) to assess sentiment in tweets, press headlines, and even Reddit posts to forecast market shifts.
According to recent insights from IBM Research, this kind of sentiment analysis can uncover public anxieties around inflation or economic instability well before such concerns appear in official statistics. In the context of ECB forecasting, tracking social media can reveal shifts in public or investor confidence—factors that influence central banks as they weigh policy options.
Take an example: When inflation unrest spiked in Germany last year, AI models trained on Twitter sentiment correctly signaled an increased likelihood of the ECB tightening rates—in contrast to a downbeat bond market. That’s a level of intuition humans couldn’t replicate at scale.
Transparent Yet Complex: Ethical Considerations in Algorithmic Forecasting
As more hedge funds and banks adopt these sophisticated models, ethical questions are surfacing. Who audits the data that feeds these predictions? Can central banks be influenced by forecast errors amplified by machine consensus?
The Financial Times raises concerns about model transparency. Many of these algorithms operate as black boxes, using neural networks that retrain continuously, making it difficult for regulators or even developers to explain how specific predictions were generated.
Moreover, integrating sensitive data—such as consumer financial behavior or private communication patterns—raises serious privacy issues. How do we ensure that in our quest for predictive mastery, we don’t cross ethical red lines?
Tools of the Trade: How These AI Models Work
The technology stack behind these systems is a mix of modern machine learning techniques—decision trees, ensemble learning, and deep neural networks. Resources on Wikipedia detail how supervised learning allows models to improve over time by measuring past prediction success against actual ECB decisions.
Financial institutions are also increasingly relying on platforms like Coursera to train analysts in deploying these tools. Courses in AI-based financial modeling and algorithmic trading give professionals a leg up in a competitive landscape.
Will AI Replace the Human Analyst?
Not entirely. Experts like Dr. Andrew Ng frequently emphasize the complementary power of AI—not as a replacement but as a tool for accelerating insight. While machines can detect patterns, humans still play a key role in interpreting the data and managing nuance and unpredictability in geopolitics and public policy.
Still, roles are shifting. Financial analysts today are less data gatherers and more AI interpreters—combining domain expertise with an understanding of model behavior and limitations.
Looking Ahead
The days of relying purely on intuition—or last quarter’s numbers—are fading fast. With AI transforming how analysts forecast central bank moves, especially those as pivotal as the ECB’s, the financial world is entering a new era of precision and speed.
But the question remains: As algorithms get better at modeling human behavior, will central banks start reacting to the forecasts themselves? In short, can AI predict a future that’s already watching itself unfold?
In the search for financial foresight, what’s clear is that machines aren’t just helping us understand the economy—they’re starting to shape it.
Conclusion
If AI can now forecast central bank decisions with uncanny precision—sometimes better than the very institutions it observes—who’s really steering the wheel? The more financial markets rely on machine-generated predictions, the more likely it is that central banks could start responding not just to data, but to the models interpreting it. This creates a feedback loop where decisions influence forecasts, and forecasts influence decisions—a paradox that challenges our very idea of independent policy.
We’re no longer just using AI to understand complex systems; we’re beginning to design systems that behave in response to how they are understood. In that light, forecasting becomes a form of quiet power—one that blends human intent with machine inference.
What happens when this power subtly shapes not just economic outcomes, but the behavior of the institutions themselves? In watching the economy, our algorithms may become part of it.
The real question isn’t whether machines can predict the future—it’s whether they might start to shape it.