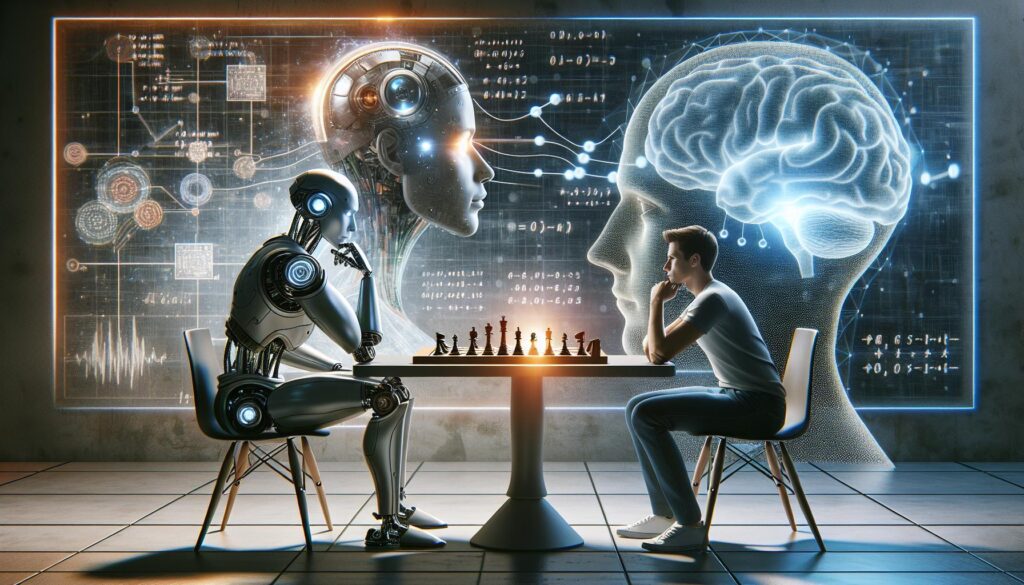
A self-driving car faced with an unexpected detour because of a spontaneous street festival might halt awkwardly or take an inefficient route. A human driver, in contrast, might roll down the window, ask for directions, or use contextual cues like foot traffic to find a better way. For all their speed and precision, smarter AI systems still falter in situations that demand common sense, flexibility, or moral judgment.
Despite rapidly advancing capabilities, artificial intelligence continues to hit roadblocks where human reasoning thrives. While AI can process petabytes of data in seconds, it struggles with uncertainty, ethical dilemmas, and decisions grounded in lived experience—things most people handle daily, almost instinctively.
The Data Cruncher vs. the Detective
Think of AI as an incredibly fast number cruncher. It’s built to optimize—identifying customer behavior patterns, analyzing medical scans, detecting fraud, and more. Platforms outlined in this Coursera breakdown of data science vs. machine learning show how algorithms can automate decision-making by relying on statistical relationships and historical patterns.
But strip away the structure, and cracks appear. Say a hospital AI must decide which patients get critical care beds during a resource shortage. It may prioritize based on survival metrics, but it won’t weigh cultural values or family dynamics the way a physician might. As explained in this University of Texas write-up on AI vs. human reasoning, real-world decisions often blend hard data with intangible factors like empathy, ethical responsibility, and social impact.
That’s where people still have the edge.
Why Pattern Recognition Isn’t the Same as Understanding
According to research from INFORMS, AI reasoning today—no matter how “smart”—isn’t reasoning in a human sense. Algorithms match patterns to known outcomes. But humans build and revise mental models based on theories, values, and intuition. We ask “why,” not just “what’s similar.”
A good example? Legal judgments. AI can analyze precedent and statutes faster than any lawyer. But complex legal cases often hinge on moral nuance or societal impact. Would you trust an AI to weigh justice in a child custody battle? Or factor in cultural context in a refugee asylum case? These are scenarios where human cognition, with its blend of experience and theory-based logic, becomes essential.
A recent World Economic Forum article asked, “In a world of reasoning AI, where does that leave human intelligence?” The answer might be: right at the intersection of oversight and creativity.
A New Kind of Partnership
The future isn’t about human vs. machine—it’s about how they work together.
Picture AI tools that tackle structured tasks quickly—like filtering job applications by skills or highlighting anomalies in financial data. Meanwhile, humans step in for decisions requiring abstraction, empathy, or out-of-the-box thinking. This kind of division of labor could enhance productivity and ethics, especially in industries like healthcare, finance, and education.
Machine learning’s role is growing in this arena, automating more of the raw analysis that formerly ate up human hours. This clears the way for people to focus attention where it’s most valuable. AI’s real potential lies in enhancing—not replacing—human intelligence.
Consider AI-assisted scientific research. Tools that once merely crunched numbers are now helping generate hypotheses and model experiments. But it still takes a human mind to question underlying assumptions, reinterpret results, and decide what’s worth exploring next.
Limitations Spark Innovation
The limitations of AI may be frustrating—but they also point the way forward. Hybrid systems that combine symbolic reasoning (how humans think) with sub-symbolic learning (how algorithms learn) offer exciting possibilities. Research published in Frontiers in Artificial Intelligence has shown early promise in integrating both methods to make machines better at handling unstructured or ambiguous information.
Still, there’s a long way to go before tech mimics—or complements—human thinking at scale. Ethics, creativity, and resilience are just a few areas where machines lag us, sometimes dramatically. As explored in this Sunscrapers guide to AI fundamentals, understanding these gaps is key to building responsible, useful AI.
What Comes Next?
AI is smarter than ever, but it’s not wiser. For every algorithm that spots cancer faster or plans a more efficient delivery route, there’s a gap in moral reasoning or intuition that only humans can fill.
So where does that leave us? Not replaced—just refocused. As AI gets better at the technical heavy lifting, it’s up to people to make sense of the bigger picture.
And that might be AI’s greatest contribution: giving us more room to think.
Because in the end, intelligence isn’t just about answers. It’s about knowing which questions are worth asking.
Conclusion
If machines can outpace us in speed, memory, and logic—yet still miss the essence of wisdom—what does that say about what intelligence truly means? As we race to build algorithms that mirror the brain, perhaps the real challenge is to understand what makes the mind more than its circuits: the ability to navigate uncertainty, weigh values, and reshape the rules when they no longer serve us.
In this rapidly shifting landscape, maybe the power of AI isn’t in thinking like us, but in forcing us to think more deeply about ourselves. What choices do we entrust to machines—and what do we reserve for the parts of being human that data can’t decode? These aren’t just questions for engineers, but for anyone imagining the kind of world we want to live in. Because as we teach algorithms how to learn, we’re also teaching ourselves what we refuse to forget.