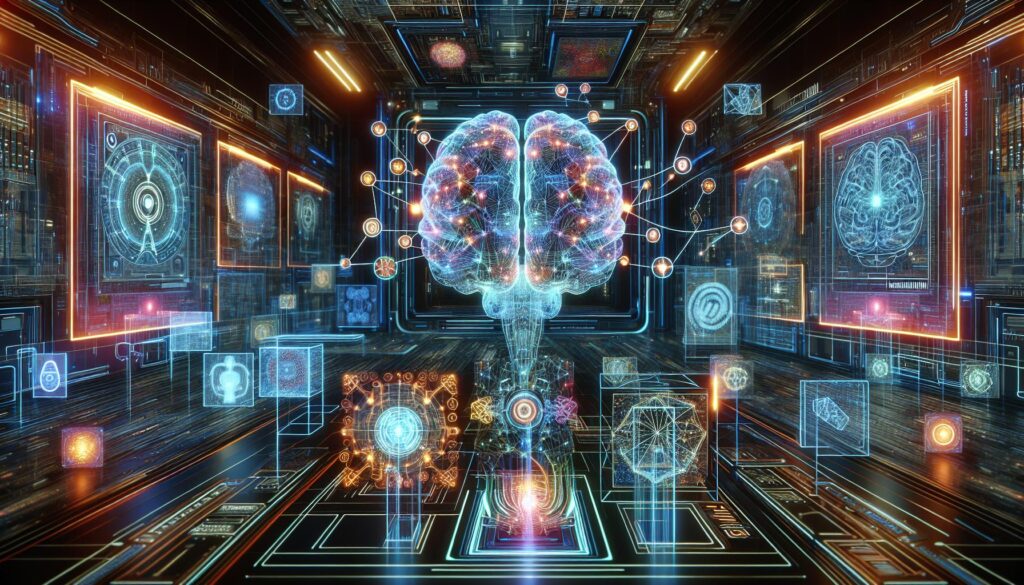
In March 2025, the ARC Prize Initiative introduced a new challenge that has AI researchers buzzing: the ARC-AGI-2 benchmark. Unlike traditional AI tests that rely on massive datasets and pattern recognition, ARC-AGI-2 focuses on something AI still struggles with—general reasoning and adaptability. The benchmark is designed to be simple for humans but incredibly difficult for machines, revealing the current limitations of artificial intelligence.
But here’s the twist: The competition surrounding ARC-AGI-2 isn’t just about building smarter AI—it’s about building AI that can learn and generalize like humans, an ability that remains elusive even for cutting-edge systems like OpenAI’s GPT-4o or Google DeepMind’s Gemini. Could this be the next step toward true artificial general intelligence (AGI)?
Smarter AI Means Thinking Beyond Training Data
Most AI models today, from language processors like ChatGPT to image generators such as Midjourney, excel at identifying patterns within massive datasets. They generate stunning text, images, and even code, but their biggest limitation is that they don’t truly understand new problems the way humans do. They rely on absorbing enormous amounts of pre-existing information rather than creatively solving unfamiliar challenges.
That’s exactly what ARC-AGI-2 aims to change. Developed as part of the ARC Prize 2025 competition, this test challenges AI systems with problems that require them to invent new solutions—not just retrieve or recombine learned patterns. It’s an approach pioneered by Google researcher François Chollet, who argued in “On the Measure of Intelligence” that true intelligence isn’t about memorization—it’s about efficient problem-solving in novel situations.
For example, consider a puzzle where a series of simple colored shapes transform based on an unseen rule. A human child might quickly understand that “red squares always turn blue” just by seeing a few examples. But for AI, which typically needs thousands—or even millions—of training examples, this kind of reasoning remains a significant challenge.
Why Open-Source AI Innovation Could Be the Key
Perhaps the most intriguing part of the ARC Prize 2025 competition is its open-source focus. In a world where tech giants like OpenAI and Google are increasingly keeping their cutting-edge AI models private, this initiative encourages researchers to share their findings, fostering innovation at a faster pace.
An open, transparent challenge like ARC-AGI-2 allows smaller research teams and independent developers to compete on equal footing with industry giants. This could lead to breakthroughs in AI reasoning capabilities, as fresh perspectives often provide unexpected solutions. And with $1 million in prize money on the line, the competitive spirit is strong.
Tech experts suggest this could also influence government AI funding, as policymakers seek to support advancements that enhance AI’s practical intelligence rather than just its brute-force computing power. According to an article published by Artificial Intelligence News, ARC-AGI-2 represents a critical shift away from data-heavy AI and toward efficiency-driven learning models.
The Road to AGI – Testing More Than Just Raw Power
Many experts argue that AI has already surpassed human capabilities in narrow tasks. Systems like AlphaGo, which defeated the best human players in Go, or OpenAI’s Dota 2 bot, which dominated professional esports, are clear examples of AI excelling in specific domains. But these systems still fail spectacularly when taken out of their designed environments.
That’s where domain-general reasoning comes in. An AI capable of smoothly switching between different cognitive tasks—from solving abstract puzzles to crafting creative text—would mark a true step toward AGI (History of Artificial Intelligence). This is what ARC-AGI-2 is testing: Can AI adapt when faced with unfamiliar, human-like reasoning challenges?
Industry experts believe we are still years—if not decades—away from achieving AGI. But the ARC Prize is forcing the research community to think differently. By emphasizing efficiency in learning, open collaboration, and fair competition, this initiative may accelerate incremental breakthroughs in AI cognition, potentially reshaping our understanding of intelligence itself.
The Future of AI: Collaborating Toward Smarter Machines
As the ARC-AGI-2 challenge gains traction, it could help bridge the gap between brute-force AI and true human-like reasoning. If an AI system can master this benchmark without relying on massive training datasets, it would be one step closer to solving problems the way humans do—by thinking, not just memorizing.
So, what happens next? If a team successfully cracks ARC-AGI-2 in the 2025 competition, it could lead to AI systems that learn as flexibly as human minds, capable of tackling brand-new scientific, medical, or engineering challenges. Or, if no AI system comes close to solving it, it could reveal just how far we still have to go in the race to AGI.
Either way, ARC-AGI-2 is setting the stage for what could be one of the most significant AI breakthroughs yet. Will this test finally expose artificial intelligence’s ultimate weakness—or prove that AGI is closer than we think?
The ARC-AGI-2 challenge represents a groundbreaking shift in AI research, forcing systems to confront problems they can’t simply memorize their way through. By prioritizing true reasoning and adaptability, this initiative could push the field beyond the current limitations of deep learning and pattern recognition. For tech enthusiasts and AI researchers alike, this is a pivotal moment—one that could shape how we define and pursue artificial general intelligence in the years ahead. Whether ARC-AGI-2 exposes AI’s ultimate weakness or sparks a new wave of breakthroughs, its impact on the future of machine intelligence cannot be overstated.
As this competition unfolds, it will be fascinating to see whether AI can finally break free from its dependence on training data and truly “think.” If successful, these advances could revolutionize fields like science, medicine, and engineering—bringing us closer to machines that learn and reason like humans. To dive deeper into this evolving landscape, check out MIT Technology Review’s latest insights on AI reasoning. And don’t forget to follow AlgorithmicPulse for more updates—share your thoughts in the comments and let us know: Do you think AI will pass the ultimate intelligence test? 🚀